We constantly hear bold hyping claims: “AI will replace us all,” “Expertise is no longer a barrier,” “We already know how to create AGI,” and so on. Influenced by media and public expectations, many people perceive potential or projected technologies as if they already exist.
In this article, we’ll break down what AI can actually do today and categorize its capabilities into four roles: Engineer, Analyst, Storyteller, and Assistant. We’ll also explore how tasks are currently distributed across these categories and predict the changes we might see in the upcoming years.
Next, we’ll dive into the technologies behind AI and explore their maturity using the Wardley Map, covering both classical machine learning and generative models. Special attention will be given to Retrieval-Augmented Generation (RAG), one of the most practical technologies for business.
Finally, we’ll address two key questions:
1. How do you know when it’s time for your business to adopt AI?
2. How do you choose the right machine learning method for specific tasks?
A typical business request
Clickbait headlines have shaped a common business inquiry: “We want to experiment with AI. We have a budget of X. If we train AI on all our data, what will it be able to do?”
In response, we often hear technoskeptics say, “AI is just a trendy hype. Nothing has really changed. A year or so, and people will forget about it like they did with blockchain. Following trends is pointless.”
The former stance feels unrealistically optimistic, while the latter unreasonably dismissive. That’s why it’s important to take an systematic approach:
- What’s actually available right now?
- What kinds of tasks can machines handle, how well they do it and how much would it cost?
- Where is the technology heading in the next couple of years?
In this article, we’ll explore the AI landscape from a practical perspective, focusing on what actually works today and what remains just a promising idea.
Now, not later — what can AI do today?
While the hype around AI is relatively recent, machine learning has been solving practical problems for quite a while.
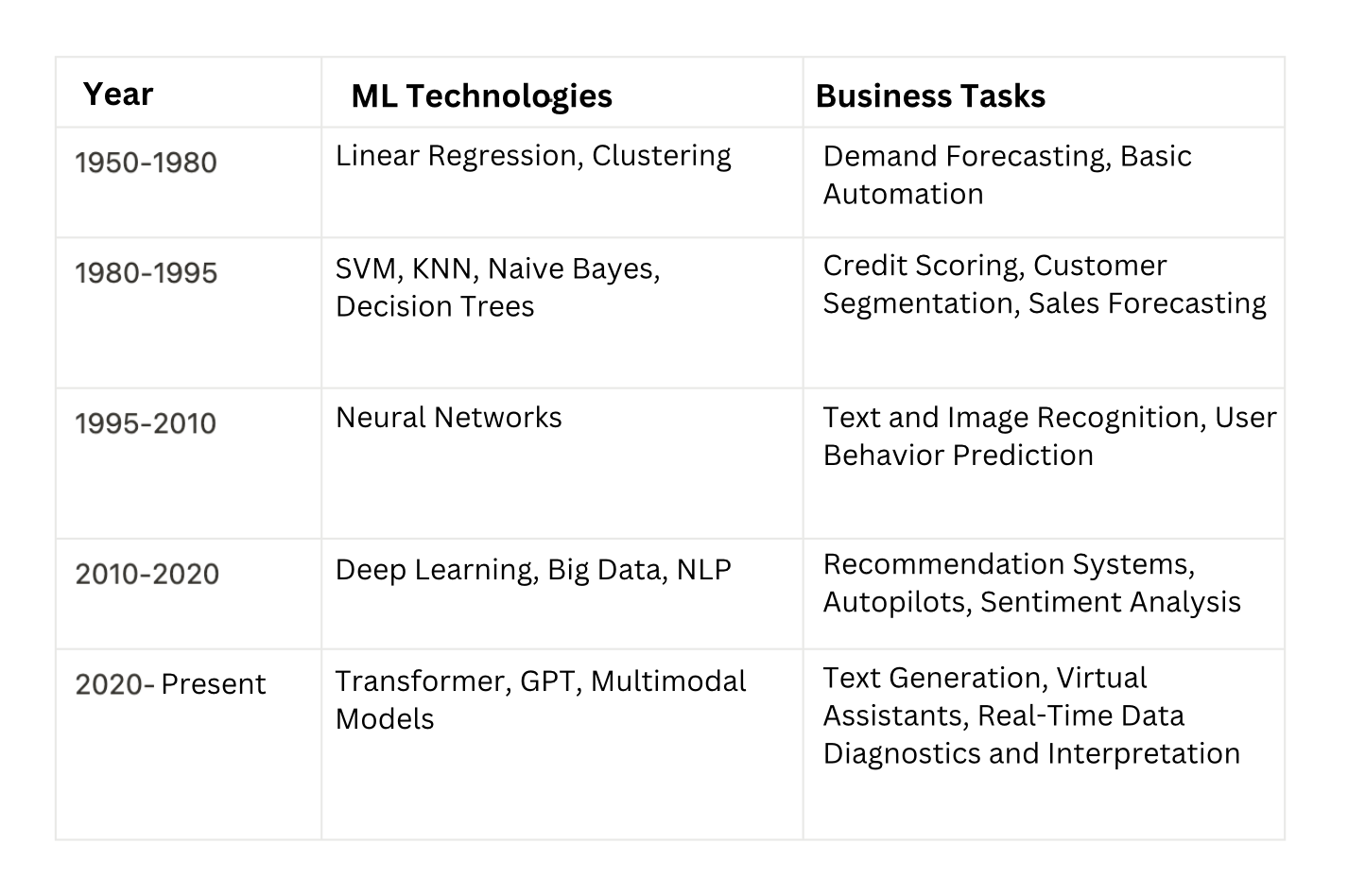
Before the rise of transformer models, machine learning methods were mostly limited to analysis and classification tasks. These models handled problems like “identify the object” or “predict the next purchase,” which had practical but fairly predictable applications.
The real breakthrough came with the introduction of transformer architectures like BERT, GPT, and their counterparts. It were transformers that enabled the shift from analysis to generation.
Generative models don’t just analyze or classify existing data, they can create new data as well. This ability has expanded the business applications of machine learning, unlocking automation opportunities in: Finance, Marketing, Design, Sales, and etc. In short, almost every department of a modern company.
Despite their power, generative models are still statistical systems. They are based on probabilistic patterns in data, not on logic. This creates important limitations:
- They operate within the scope of “public knowledge” from their training data.
- They lack domain-specific expertise–they don’t understand rules, methodologies, and can't interpret formal knowledge systems.
If you want a model to truly understand a niche field, that expertise has to be manually embedded.
Thus, If we map today’s AI tasks along two key axes “Analysis vs. Generation” and “Public Knowledge vs. Specialized Knowledge” we get four major categories of applications. Those categories can be called: Engineer, Analyst, Storyteller, and Assistant.
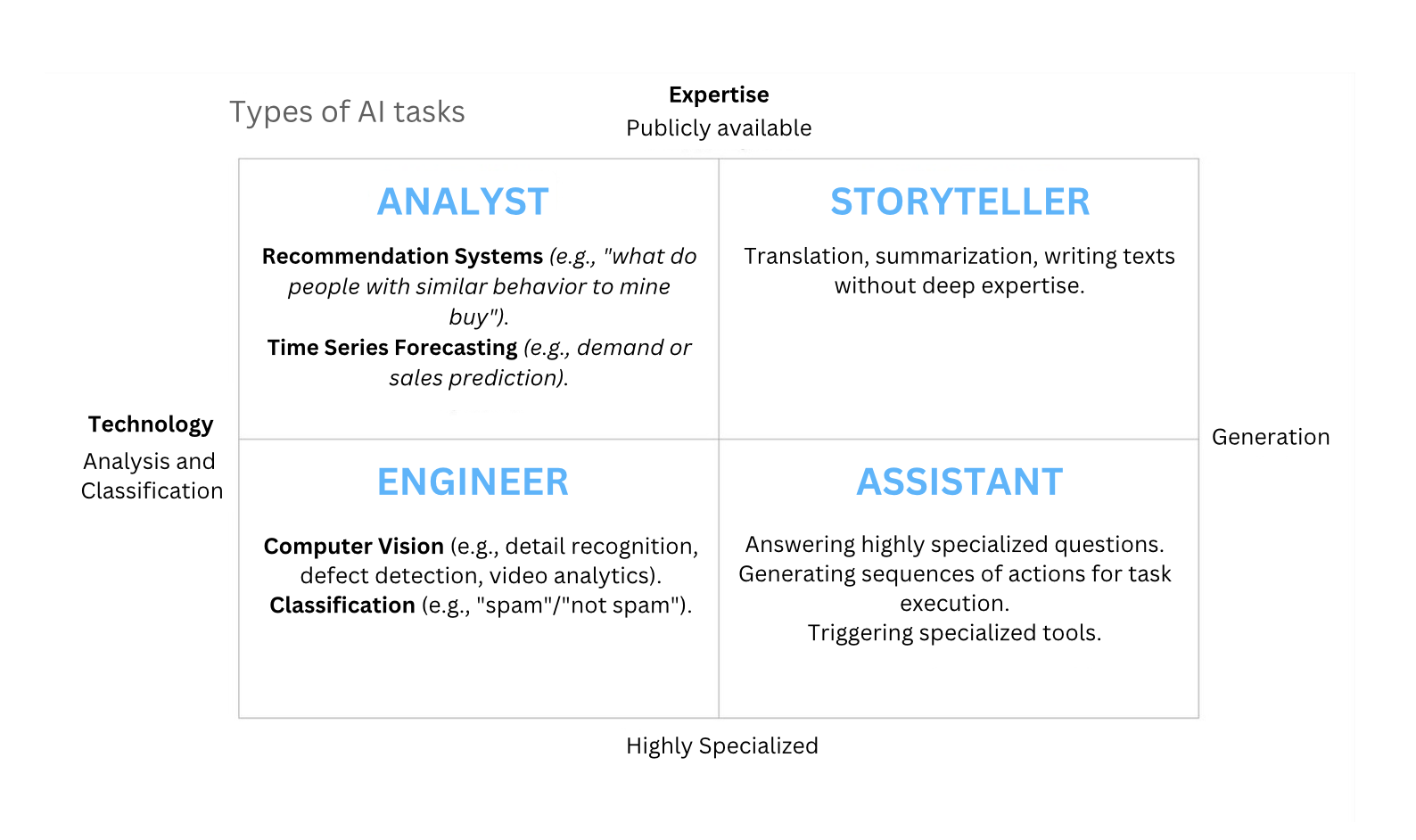
Engineer
This category covers tasks that require human expertise for data preparation: primarily in computer vision and classification.
Computer vision systems can, for example, detect defects on production lines, analyze product display quality in stores, or identify objects in videos.
However, for such models to work effectively, a large amount of labeled data is required. Human experts manually create datasets, for example, by classifying defects in cargo photos or tagging products in a store. The more extensive and accurate the data, the better the model performs.
Examples:
Analyst
This category covers tasks based on classical machine learning algorithms and largescale statistical data.
An example is recommender systems or time series forecasting (predicting sales, demand changes, or traffic trends).
These systems don’t require human experts but they rely heavily on continuous feedback. It is crucial to regularly retrain the model on new data.
Examples:
Storyteller
Generative models like ChatGPT kickstarted a new class of tasks where AI doesn’t just analyze information, but creates new content. However, as we mentioned earlier, this process is based on statistical patterns in data, not on logic or deep expertise.
As a result, they handle tasks like translation, summarization, and text generation well, but their output can vary significantly from one run to another. Moreover, these models are prone to hallucinations–they may invent nonexistent events or facts.
Working with such systems requires an understanding of their limitations. Human involvement is mainly needed for writing prompts–requests that guide the generation in the desired direction. However, prompts can only capture simple knowledge, while deep industry expertise remains out of reach.
Assistant
AI Assistants combine generative technologies with specialized knowledge. They don’t just generate text they operate within an expert defined logic, can answer domain specific questions, plan actions, and interact with other systems.
Creating such assistants requires active involvement from human experts. They define the methodology, embed relevant knowledge, and set the boundaries within which the AI operates.
Examples:
Task distribution between "Engineer," "Analyst," "Storyteller," and "Assistant": current state and what will change in a few years
Based on my observations, which are not based on quantitative analysis but rather on daily communication with clients, the current task distribution across these quadrants is as follows:
- 40% – Engineer. This includes computer vision tasks for robots, warehouses, retail, and industry.
- 30% – Analyst. The main pool of tasks is related to recommendation and predictive systems for eCommerce, marketing, and finance.
- 20% – Storyteller. Generative models are actively used for content creation, translation, and summarization, particularly in areas where errors are acceptable, such as marketing and creative fields.
- 10% – Assistant. The main demand currently comes from highly specialized fields like medicine, law, technical support, and education, where it’s critical not just to generate text but also to ensure its accuracy.
What Will Change in the Next Few Years?
The growth of data volumes, technological breakthroughs, and increased trust in generative systems will lead to a redistribution of tasks among these categories.
According to my prediction:
- The share of engineering tasks will likely decrease slightly (from 40% to 30%) as some simple computer vision tasks will shift to multimodal LLMs, making them much simpler.
- The share of analytical tasks will likely decrease slightly (from 30% to 20%). Some tasks related to search and recommendation systems are already being handled by LLMs.
- The share of generative tasks (Storyteller) will likely increase (from 20% to 25%), as the quality of language models continues to improve and their applications expand.
- The most noticeable growth (from 10% to around 25%) will be in the AI Assistant category, driven by two key factors. Firstly, AI Assistants are starting to take on simple tasks from other categories. Secondly, AI Assistants are becoming central management points, interacting with other models–such as requesting data from recommendation systems or accessing knowledge bases to verify information.
What technologies power AI?
In any rapidly evolving field, there are technologies at different stages of maturity: some are still in the experimental or proof of concept phase, others are used in early custom implementations, and some have become massmarket products or are as common as electricity.
The newer the technology, the more “raw” it is, and the higher costs for implementation and greater business risks it requires. However, if the implementation is successful, it can provide a competitive advantage.
But as the technology matures, what we spent a lot of money on today will become much cheaper and more accessible to our competitors within a year. So, even if you manage to successfully adopt cutting edge technology, you need to act quickly to make the most of the competitive advantage it provides.
On the other hand, if a highly mature technology is available on the market, not being aware of it or not using it means falling behind competitors–paying significantly more where others operate cheaper and more efficiently. It’s like not using the internet or electricity.
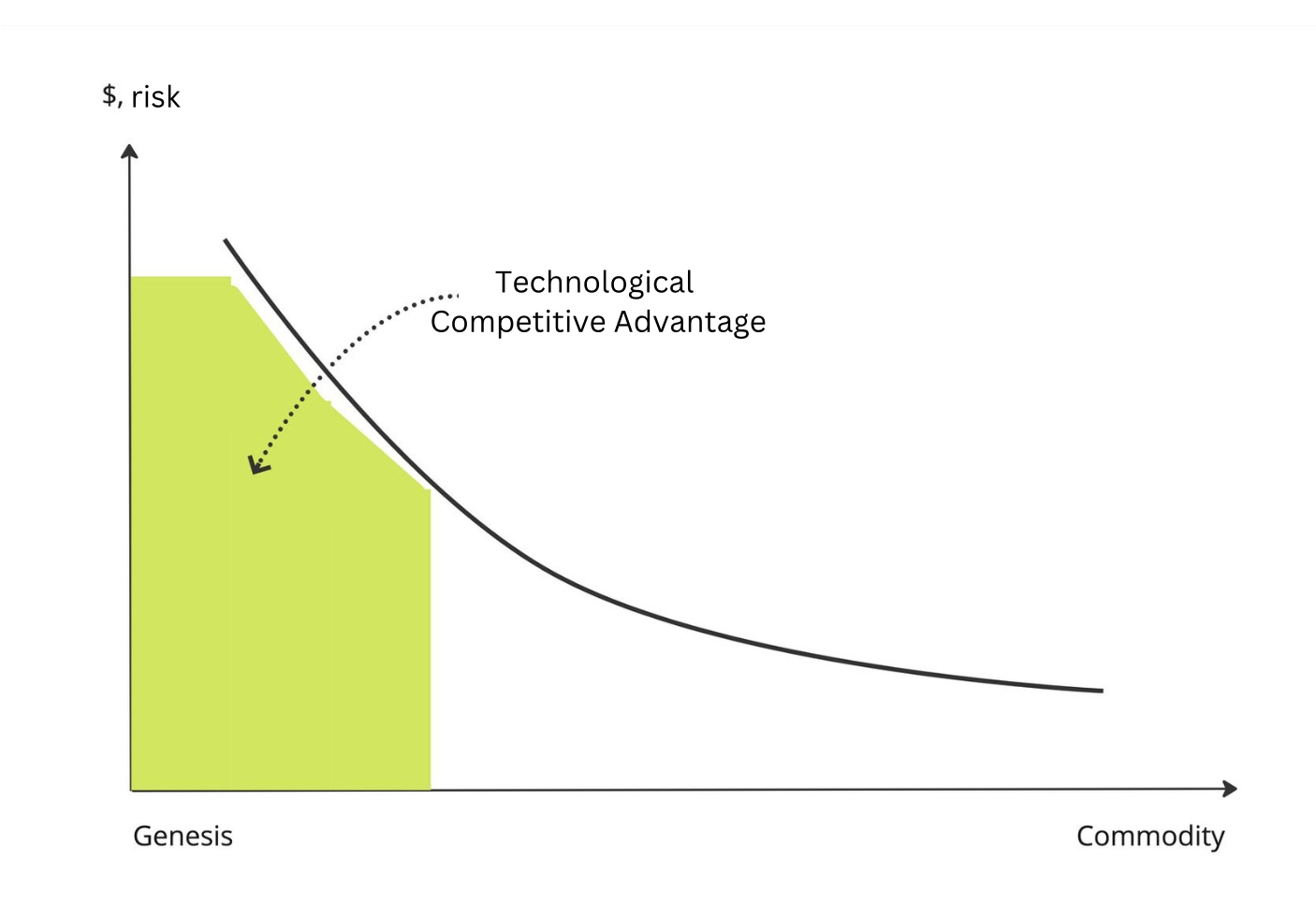
So, let’s take a look at the maturity of key machine learning technologies, both "classical" and "generative." To keep things organized, we’ll use a Wardley Map, a diagram where technologies are positioned horizontally based on their maturity and vertically based on their visibility to businesses.
Infrastructure technologies are marked with a diamond. They are crucial for the development of business relevant applied technologies (marked with a circle), but are almost invisible to businesses, which is why they’re placed at the bottom of the map.
Wardley map for "Classic ML"
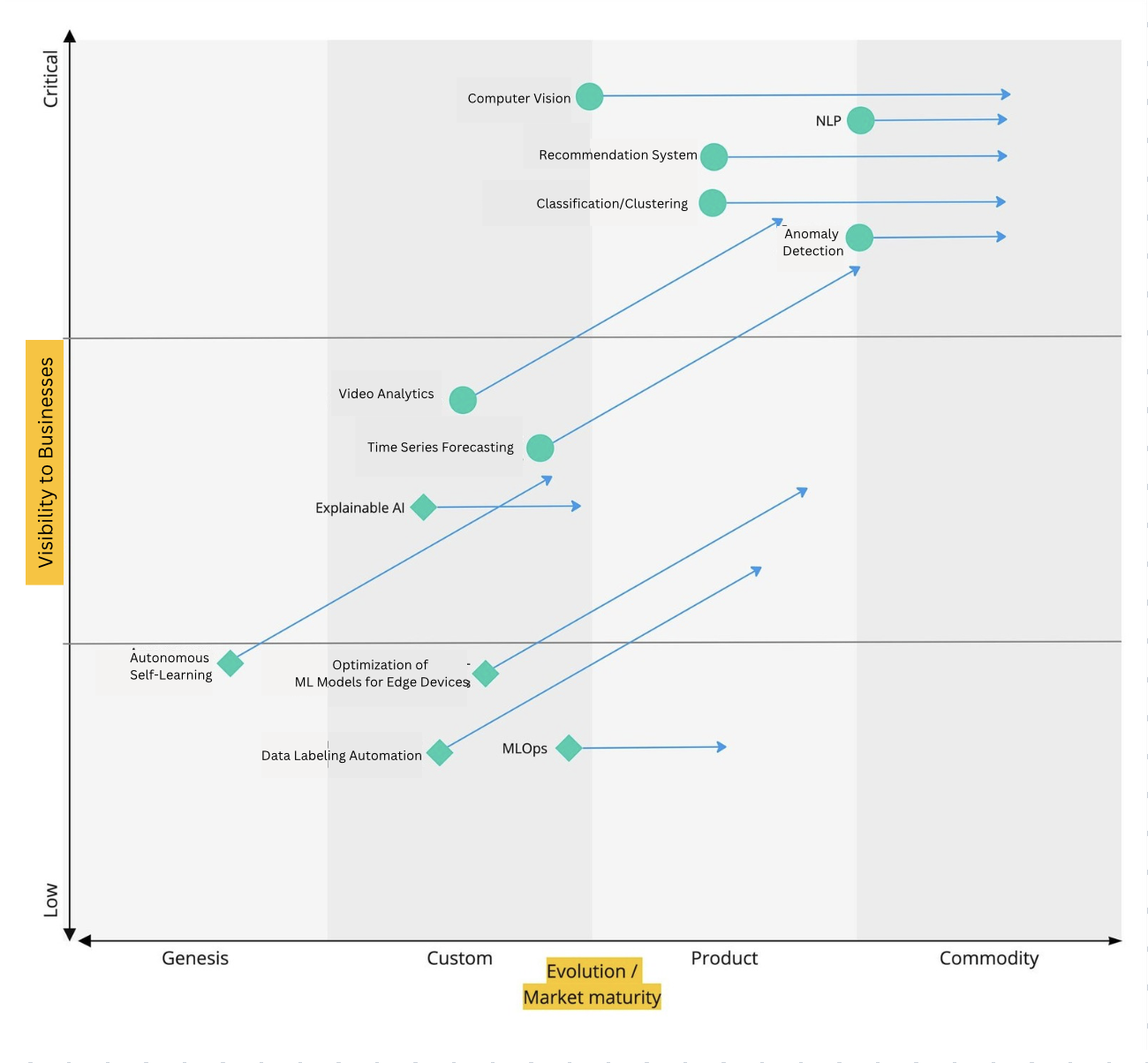
Let’s look at all the parts.
The situation overall: despite common expectations, ML models do NOT get better over time.
There’s a common expectation that you can simply run ML models, and they will automatically learn from incoming data, gradually getting better over time.
In reality, retraining ML models with new data is a manual, timeconsuming process. The idea of ML systems learning without human help is still more of a research topic than something practical. Also, adding more data doesn’t always improve the results – data quality and proper labeling are key.
Based on this expectation, most of the progress in "classical ML" is now focused on developing tools that make it easier to label data, train ML models, and explain to models what you need from them. For example, you can show a computer vision (CV) algorithm a few examples of what damage on a box looks like, and it quickly understands the pattern.
Technologies for autonomous selflearning in ML models are just starting to develop. For now, retraining ML models still requires a manual approach and complex technical solutions.
Computer Vision and video analytics: models don’t understand scene context
Computer vision technologies are on point when it comes to recognizing anything in static images. The quality of detection algorithms is so impressive that some applications are truly fascinating, like identifying LEGO pieces or detecting packaging defects in a warehouse.
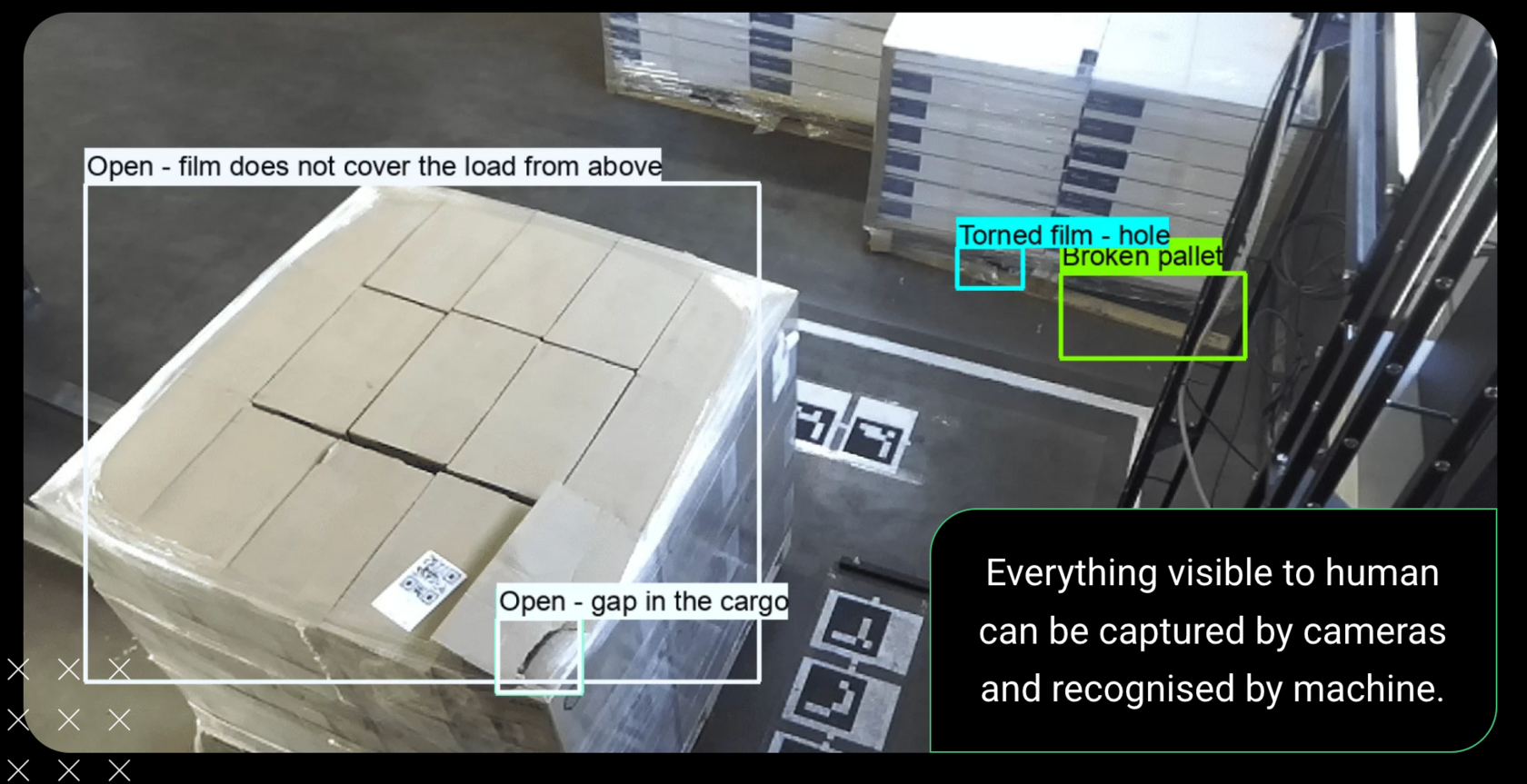
What Computer Vision Algorithms Can’t Do Yet:
They struggle to understand the full context of a scene. It’s hard for them to handle dynamic environments with many moving objects. They also have trouble when familiar surroundings change – for example, if a warehouse floor is repainted, the CV models will likely need retraining.
Video analytics systems face the same limitations as computer vision. On top of that, for robotics and drones, video recognition has to work in realtime on the device itself. This means running on hardware with limited processing power, not relying on some killer cloud GPUs.
That said, solving these issues seems like a matter of time in the near future – and that’s without any tech optimism.
Predictive Models: not great at predicting
As Professor McGonagall once said, "Divination is a very imprecise branch of magic."
Many predictions, such as demand, prices, traffic, inventory, weather conditions, currency rates, and other timedependent parameters, are based on Time Series Forecasting.
This approach is mature and has been used in business for a long time, but it has two fundamental problems:
1. Not all data fits into time series. Many business processes depend not just on time but on complex, nonlinear interactions.
2. The data might not have enough signal for accurate predictions.
In computer vision, you can often improve model quality by adding more labeled data. But with time series forecasting, you’re stuck with the data you have. If the predictions are poor, you can either try creating new features (like thinking, “Maybe this depends on the weather–let’s add weather data”), or test different model architectures. But if predictions are still bad, there’s not much you can do.
That said, predictive models are becoming more accessible. Pretrained models, cloud solutions, and AutoML tools that automate model tuning are all on the rise. However, the core problem remains: if there’s no strong signal in the data, the predictions will be weak.
NLP (Natural Language Processing): shows impressive results thanks to Large Language Models
Overall, NLP (Natural Language Processing) is a mature technology at the commodity stage for most business applications. However, different NLP tasks vary in how advanced they are:
- STT/TTS (Speech to Text / Text to Speech). Voice recognition and transcription work great for popular languages (English, Chinese, and Spanish) but not as well for rare languages and dialects. The main challenge is computational power: realtime STT/TTS needs strong hardware. Also, synthesized voices still sound robotic.
- OCR (Optical Character Recognition). Works well for printed text but struggles with handwriting. Structured text, like tables and forms, is harder to process because models don’t fully understand the page layout. This might improve with AI based OCR systems.
- Text Classification and Clustering. Performs well in narrow fields, like spam detection. However, it requires a dataset with labeled examples for training. Fully automatic, error free classification isn’t possible yet. Using large language models (LLMs) significantly improves accuracy.
- Named Entity Recognition (NER) and Fact Extraction. Works well for simple entities like names, dates, and companies, but struggles with complex ones, such as legal or medical terms. LLMs improve NER performance, though they still need finetuning for specific domains.
- Sentiment Analysis. Works exceptionally well. With LLMs, sentiment detection has often become more accurate than human judgment.
Anomaly Detection
Used to identify anomalies in time series data (like website traffic), transactional data (such as fraud detection or accounting irregularities), and in images and videos (defect detection or motion tracking). This is a mature technology, though realtime anomaly detection is still a challenging task.
Recommender Systems: from website widgets to conversational recommendations
Traditional recommender systems (collaborative filtering, content based filtering) work well but struggle in situations with many new users or cold start problems. LLMs are starting to complement or even replace traditional systems, especially in ecommerce, where conversational recommendations in chat interfaces are becoming more common.
Business applications
These building blocks are combined to create business solutions, such as:
- Fraud Detection Systems (anomaly detection + time series forecasting + classification + clustering).
- Content Moderation (computer vision + anomaly detection + speechtotext (STT) + classification + clustering).
- Predictive Maintenance (time series forecasting + anomaly detection).
- Logistics Optimization (demand, inventory, and price forecasting)
- Advertising and Marketing Optimization (recommendations + time series forecasting + clustering).
Overall, when analyzing a business problem, it’s more useful to think in terms of specific functional “building blocks” rather than vague ideas like “AI is thinking.”
Wardley Map for "Generative ML"
The technology landscape in generative machine learning is much more dynamic. This is because its growth is largely driven by the rapid shift of large language models (LLMs) from the Genesis stage to Commodity. Right now, these technologies are at the core of most business demands for “AI adoption.”
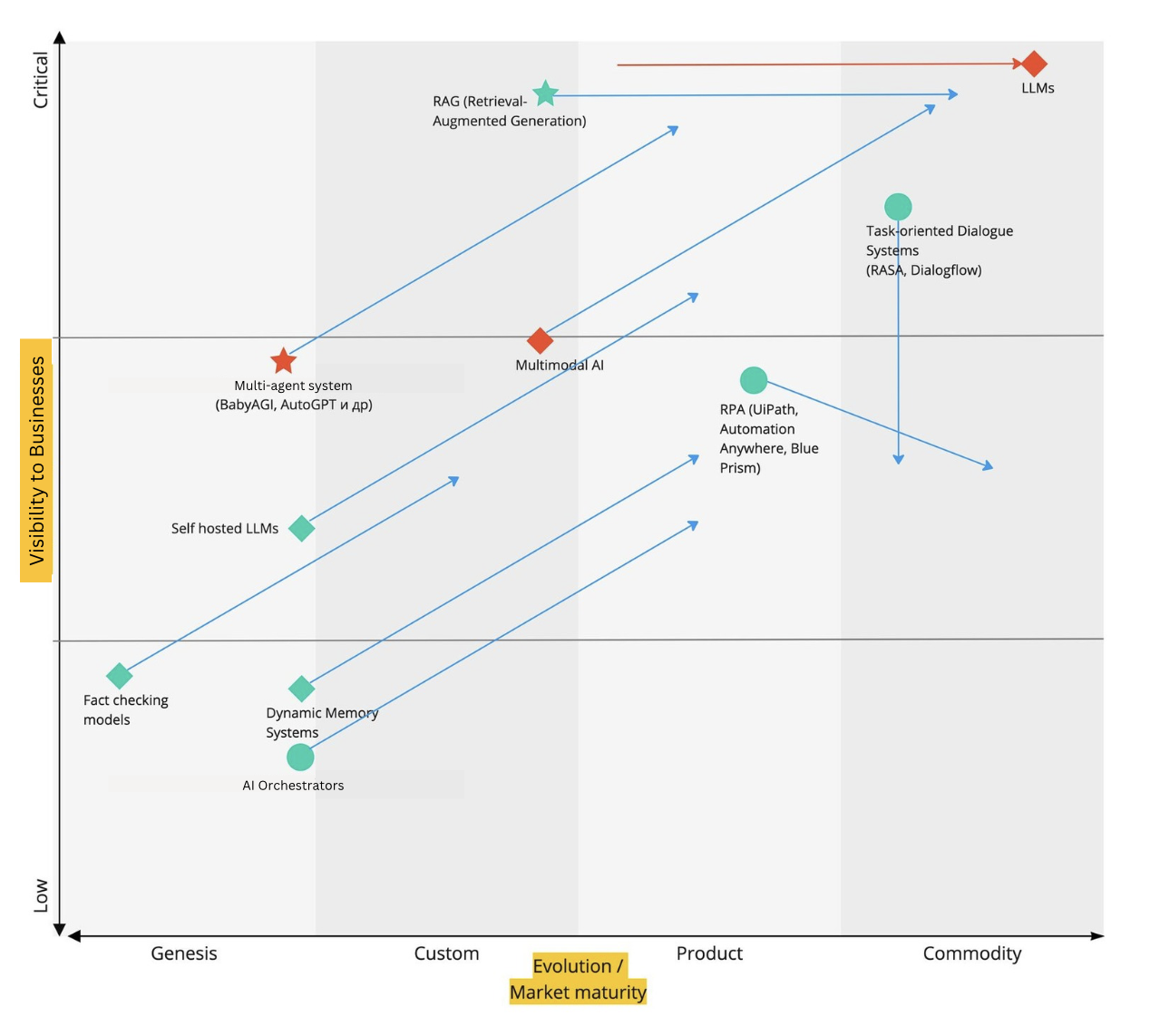
However, the majority of technologies on this map are still in the Genesis stage.
MultiAgent Systems: The Hype Engine
Multiagent systems (like AutoGPT, BabyAGI) aim to teach models how to plan, make decisions, and manage other agents. This technology fuels the popular narrative of “AI will replace everyone,” but the current reality is far more modest.
Agents are still too noisy, expensive, and unreliable. Their use cases are narrow and require strong engineering expertise. Companies with advanced ML teams are actively working on them, but widespread adoption outside of IT is still a long way off.
However, as technology advances, multiagent systems will gradually take over dialogue management, which is currently more reliably handled by taskoriented solutions like RASA and Dialogflow. Over time, traditional dialogue systems will lose their key role, giving way to more flexible and adaptive agents.
The Future of RPA: moving toward AI assistants
RPA systems (Robotic Process Automation) like UiPath are widely used to automate routine business tasks, such as document processing, working with interfaces without APIs, and performing repetitive operations.
However, the rise of AI assistants is gradually changing this landscape. In the coming years, many tasks currently handled by RPA systems will likely shift to AI assistants.
That said, traditional RPA tools probably won’t disappear. Their role will simply evolve. They’ll remain valuable where strict rulefollowing and high predictability are needed. But for tasks that require flexibility and adaptability, AI assistants will have the upper hand.
Multimodal AI: a mature technology with growing demand
Multimodal models combine the analysis of images, text, audio, and video. Today it is already a mature technology, with successful examples like CLIP, Flamingo, and Gemini.
The key advantage is that these models can merge different data types, opening up new possibilities–like analyzing images with text context in mind.
This technology is already in active use, and its maturity will continue to grow.
AI orchestrators
An AI orchestrator is a tool for building visual workflows to manage chains of requests in LLMs. Some examples of such services include Langflow and n8n. They are used when a task can’t be solved with a single prompt and requires a sequence of multiple steps. For example, the first prompt might determine the topic of a message, and the second processes the message differently depending on that topic.
As LLM usage grows in business, these tools will become more important, especially for teams looking to speed up the development of LLMbased solutions.
RAG: The Most Mature Technology on the Map
The most mature and visible technology on this map is RAG, and here’s why that matters.
RAG (Retrieval-Augmented Generation) as the most business ready technology right now
The most mature and businessrelevant technology on this map is RAG (Retrieval-Augmented Generation).
While many people have practical experience with LLMs, they’ve also noticed that LLMs give poor answers on topics outside of general knowledge.
RAG solves this problem. It’s a way to design AI assistants so they pull information not from public knowledge but from their own internal databases.
Where RAG Is Used:
- Corporate AI Assistants. When the assistant answers questions based on an internal knowledge base, not general information.
- AI Customer and Tech Support. When the AI pulls data from a knowledge base instead of relying on rigid scripts.
- Document and Reporting Automation. When the assistant uses external databases to summarize or find information before generating a response.
Such an assistant first retrieves relevant data based on the user’s query, similar to how a search engine works. Then, using the retrieved data (documents, API responses, etc.), it generates an answer, referencing specific parts of the data.
This approach has two key advantages:
- It helps address one of the biggest issues with language models hallucinations, where models generate nonexistent facts.
- It allows for embedding reasoning logic when answering questions. For example, in tech support, the assistant can check what equipment and services a user has and, based on that, suggest the possible cause of an issue.
How RAG differs from traditional search:
- Personalization. When an AI assistant gathers data to answer a question, it can search a database, query a CRM using the user’s account (to get details like connected equipment and services), or request analytics data (like the user’s support history over the past month). All this information can be factored into the response.
- Reasoning. When preparing an answer, the AI assistant can analyze the topic of the user’s query and reason through possible solutions. It can either follow a predefined decision tree or leverage the builtin capabilities of language models for common domains, such as general sales knowledge.
The crux of a RAG assistant is its data. The technology behind it isn’t complicated.
We’ve already discussed RAG assistants in a previous article and during our talk at Knowledge Conf (video).
How to know it’s time to implement AI
The worst reason to adopt AI is the "everyone's doing it, so I will too" mindset, which we often see nowadays.
All these cases where companies don’t fully understand their own business processes or what exactly they want to achieve, yet plan to throw "all the data" into AI hoping it will "figure it out on its own," usually lead to frustration and wasted budgets.
Don't be like that:
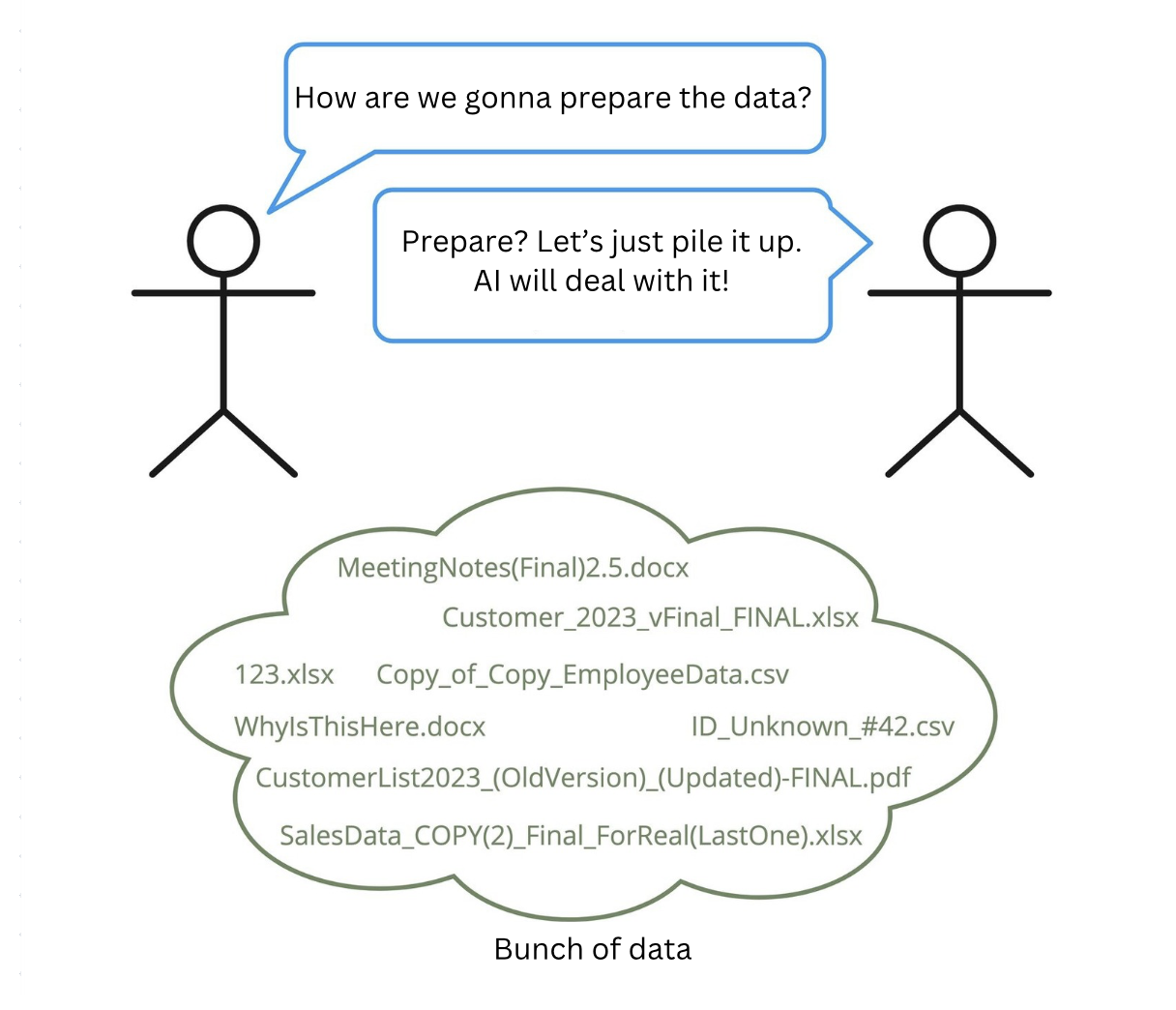
In my view, the clearest signals that it’s time to consider ML/AI automation are when you feel:
- “Our biggest problem is inefficiency.”
- “We spend too much time on this task.”
- “We lose too much time/money/patience due to errors.”
This means you have a somewhat clear business process and at least an intuitive sense of the bottlenecks that automation could address. That’s already a good starting point.
A useful rule of thumb when deciding on automation is to imagine hiring an intern instead of an ML algorithm. Think of a very smart, hardworking intern who works 24/7, doesn’t get distracted, and doesn’t make careless mistakes. But like any intern, they need clear instructions:
- What’s their task?
- What’s the input?
- What’s the expected output?
- What rules should they follow to turn the input into the output?
If the task is clear enough for a very smart, talented and hard-working intern to handle, then it can likely be automated with a combination of AI/ML methods.
How to choose the right Machine Learning/AI method for your task
Even if a business correctly identifies a process that can be automated, the AI hype often skews the focus when it comes to choosing the right solution–leading to the use of inappropriate tools.
A common mistake is trying to replace traditional tools with LLMs without any real justification. For example:
- Using GPT/LLM for Time Series Forecasting Instead of Specialized Algorithms. LLMs can't analyze time series data and don’t handle historical data like LSTM, Prophet, or gradient boosting models do. They simply memorize patterns from the training dataset rather than building true predictive models. The Right Tool: Specialized ML algorithms for time series forecasting (LSTM, XGBoost, and Prophet.)
- Relying on GPT or LLMs for niche knowledge instead of using RAG (RetrievalAugmented Generation) or standard indexbased search. LLMs don’t search databases–they generate responses based on their training data. Unlike search systems, LLMs can "hallucinate" and confidently provide inaccurate answers. Right tool: For factual search–RAG (RetrievalAugmented Generation), Elasticsearch, vector databases (Weaviate, Pinecone). For generating responses based on retrieved data LLM + RAG.
- Using generative models for structured data analysis. Applying GPT/LLMs to analyze financial reports or inventory data is ineffective. LLMs don’t perform precise numerical calculations; they attempt to "guess" answers based on statistical patterns from their training data. Right tool: BI tools (Power BI, Tableau), SQL, Pandas, or specialized ML models for data analysis.
What about DeepSeek (or any other new technology)? Isn’t it a breakthrough that changes everything?
Every new technology may seem revolutionary at first, but its real impact can be assessed systematically. One effective approach is using Wardley Maps, as we discussed in this article.
If we load these maps into GPT4 and ask it to analyze the emergence of a new technology (like DeepSeek), the model can:
1. Retrieve information about the technology,
2. Analyze its differences from existing solutions,
3. Evaluate market shifts and identify truly significant changes.
This way, instead of reacting emotionally with statements like “this changes everything!”, you can get a rational analysis that helps determine whether a new development represents a true technological leap or just another wave of marketing hype.
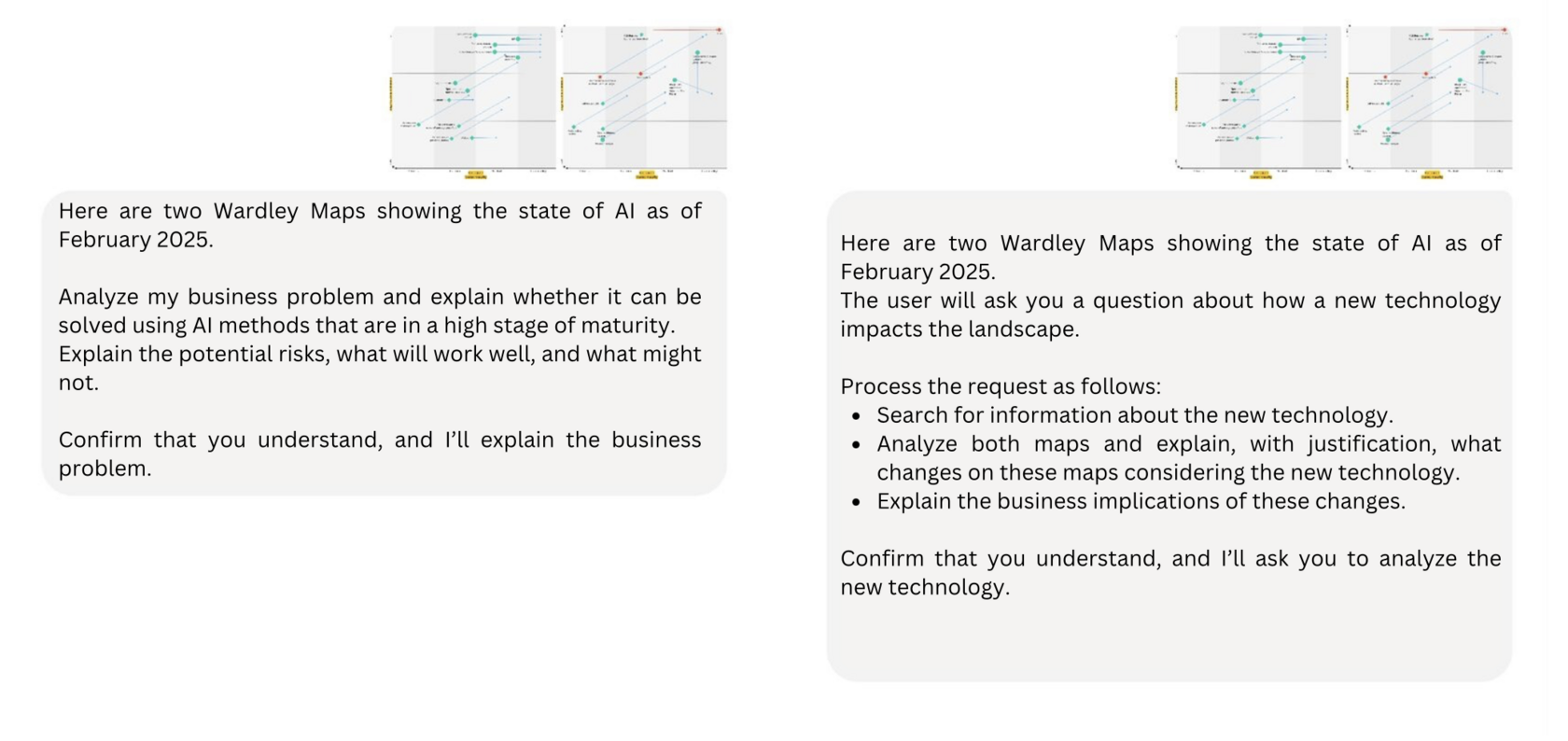
And if there’s a question about whether or not a business problem can be solved with ML/AI, you can always ask GPT. Well, at least on an initial filter level :)